Visual Analytics of Natural Language and Linguistic Data
As part of an interdisciplinary effort computer scientists and linguists at the University of Konstanz jointly develop novel ways of visually analyzing natural language for linguistic inquiry. The research questions under investigation mainly come from the linguistic fields of Typology and Historical Linguistics. The focus lies on an investigation of language features and phenomena from both cross-linguistic and diachronic perspectives. Currently, three main areas are being worked on that will be shortly described in the following.
Cross-Linguistic Comparison of Language Features
Comparing language features across different of today’s languages is key to learn more about causes and effects of language change. It can help to better understand natural language and reveal which kinds of phenomena are shared across all languages. Such phenomena can be considered rather stable over history and therefore universal. Other phenomena may, in contrast, vary heavily across languages which indicates that they are prone to change. Unfortunately, for many language phenomena the available information is sparse and imprecise, i.e. it corresponds to a rough manually constructed categorization that is only available for a subset of the world’s approximately 6,900 languages. However, there are cases where this gap in precision and amount can be filled with the support of computational means. Certain language phenomena may be approximated by deriving numerical features from texts. Those features may consist in rather complex sets of relations and therefore suitable visualizations are required to convey them to the analyst. One example for language features are vowel distributions within words, which indicate whether a language contains Vowel Harmony or uses Syllable Reduplication (see Figure 1).
High-resolution version containing 42 languages, click here.

Analysis of Geo-spatial and Genealogical Distributions of Language Features
When comparing features across languages it is of special interest to explore the geo-spatial and genealogical implications. It might be the case that only genetically related languages or languages in a specific world region share a certain peculiarity in a feature. Such findings help to hypothesize whether a certain feature is likely to be influenced by language contact or is rather likely to be inherited from proto-languages without changes. One preliminary example of plotting the geo-spatial distribution of a language feature using a distorted world map is shown in Figure 2.
For more details we would like to refer you to the corresponding paper.
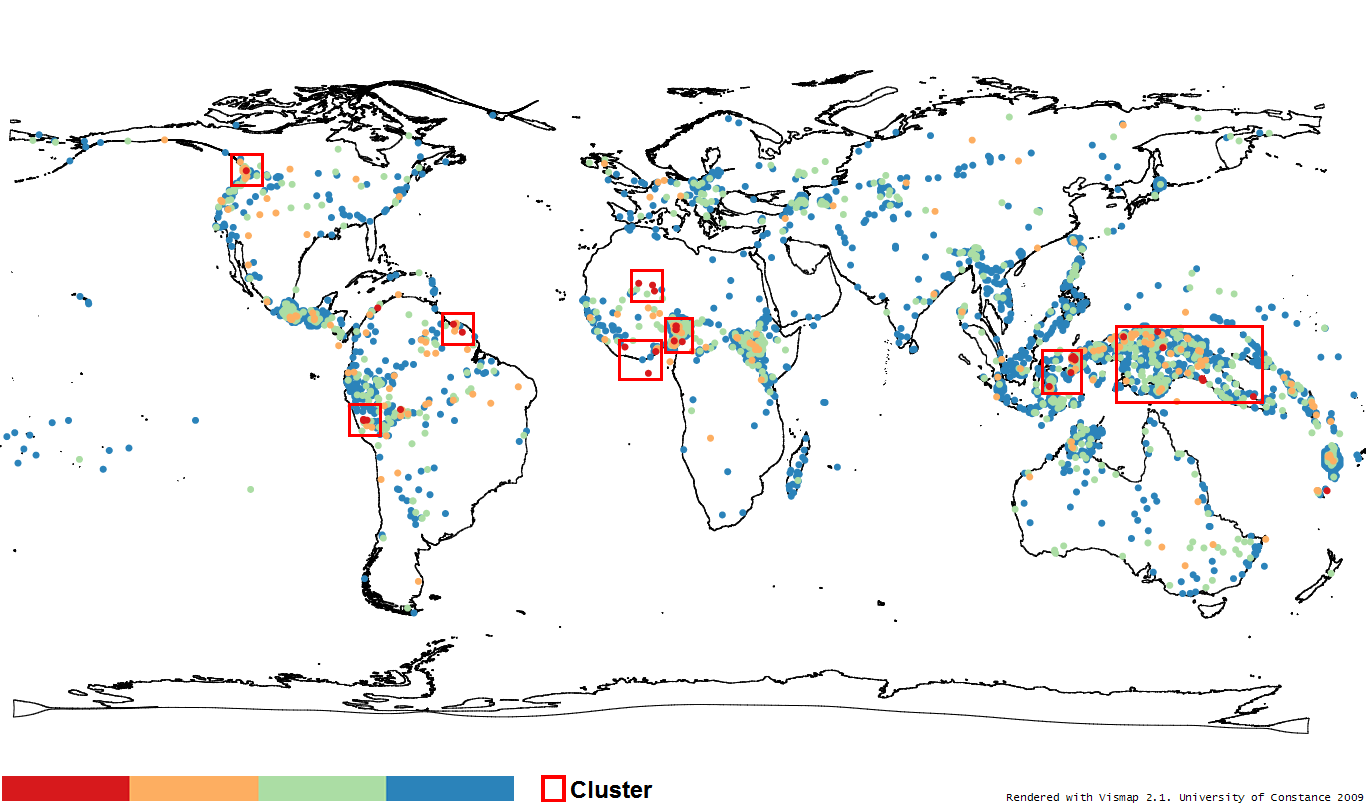
Diachronic Analysis of Lexical Semantic Change
It has been shown that different meanings of a word can be disambiguated and even induced by their context. Within this project we aim to develop visual analytics approaches that track the development of word meanings over time. The aim is to gain a deeper understanding of how lexical semantic change happens. Figure 3 shows the quantitative development of different automatically learned senses for the words “to browse” and “to surf” based on daily newspaper editions over 20 years.
For more details we would like to refer you to the corresponding paper.
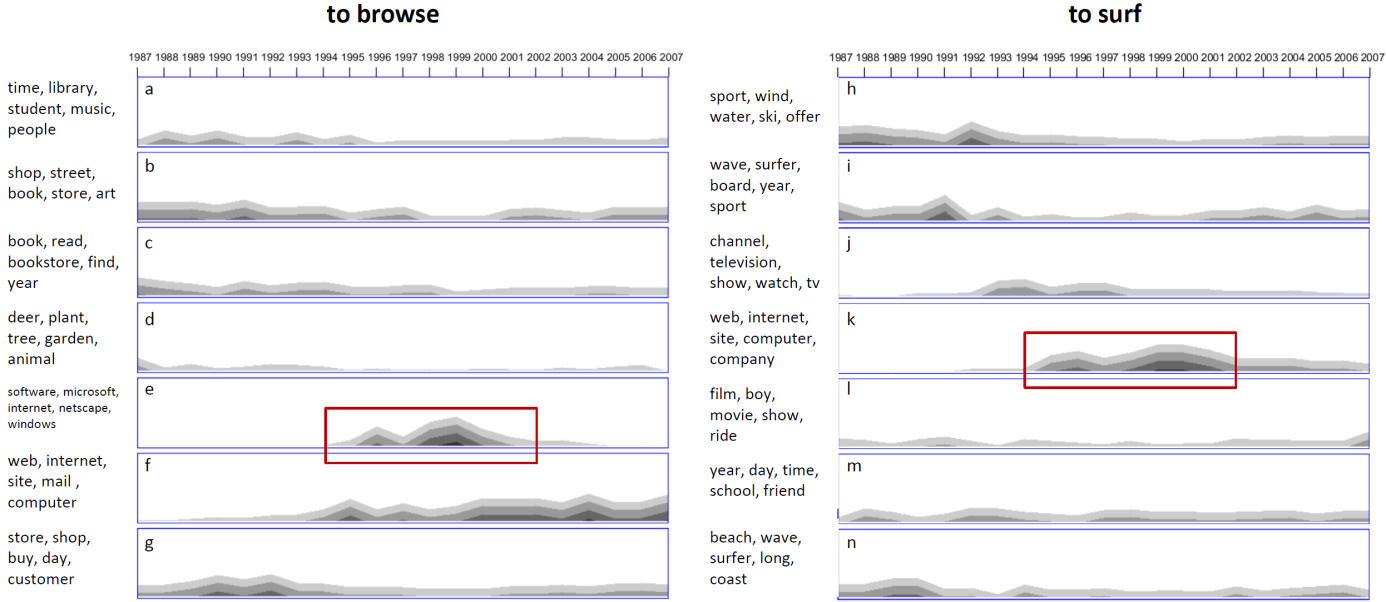
More information about this and related work can be found in the following publications.